Endometriosis Prediction by "Machine Learning"
Sep 30, 2022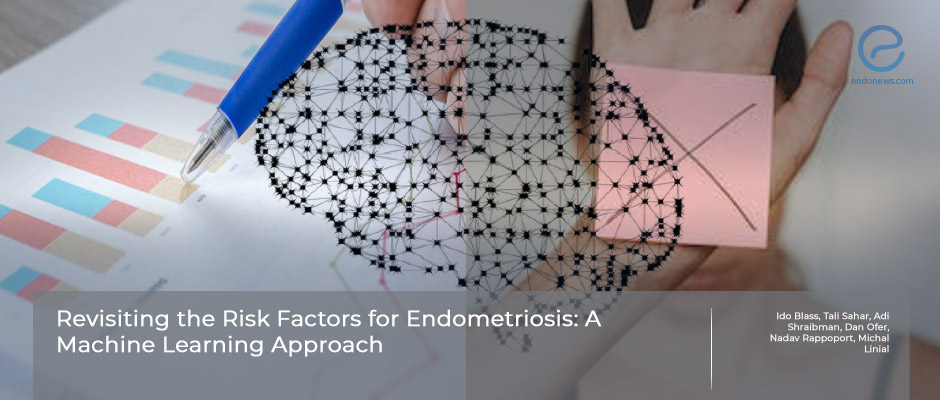
Pertinant machine learning models and their incorporation into the clinics may help to decrease the latency in endometriosis diagnosis.
Key Points
Highlights:
- Large cohorts of women diagnosed with endometriosis from the general population may provide peculiar information.
- The incorporation of comprehensive systematic models into the clinics will have an impact on diagnosis and treatment.
Importance:
- The construction of machine learning models and their incorporation into the clinics may improve the personalized approach and help to decrease the latency in endometriosis diagnosis.
What's done here:
- The aim is to develop an endometriosis model and re-estimate the known risk factors.
- A retrospective controlled study designed by Blass et al. by using the UK-biobank data evaluated 1000 variables about lifestyle.
- The data of a total of 142,723 patients and 71,088 age-matched samples were compared to 5924 endometriosis patients.
- For the predictive model, medical history, nutrition, lifestyle information, and genetic data were combined via a machine-learning model.
Key Results:
- The average endometriosis diagnosis was at 42,1 years.
- Prior to being diagnosed with endometriosis, affected women had a significantly higher number of a variety of diagnoses than the average unaffected women.
- An explainable AI tool revealed irritable bowel syndrome and the length of the menstrual cycle were informative features.
- In their machine learning framework, the genetic variants slightly contributed to the discriminatory value.
- Menarche age, smoking, and BMI were not proposed as strong indicators of endometriosis in the above endometriosis models.
Strength and limitation:
- A large number of patients and controls of different ethnicity, and the statistical assays are the strengths.
- Limitations are the inclusion of postmenopausal age data, noisy medical input, missing data, and inherent limitations of the model.
Lay Summary
To develop a predictive model for endometriosis and to re-assess the contribution of known risk factors, Blass et al. from the Hebrew University of Jerusalem, Jerusalem, Israel used the data of the UK biobank by machine learning-based models. They compared and statistically evaluated the personal data of 5924 women with the diagnosis of endometriosis to 71,088 control samples.
The UK biobank covers 500.000 participants collected from 23 medical centers across the UK. After retrospectively analyzing over 1000 variables including personal information related to female health, lifestyle, genetic variants, and medical history prior to endometriosis diagnosis, the authors applied machine learning algorithms to train an endometriosis prediction model.
The most relevant association between endometriosis and previous disorders was found as irritable bowel syndrome and menstrual cycle disorders. Prior to being diagnosed with endometriosis, affected women had a significantly higher number of a variety of diagnoses than the average unaffected women.
The researchers demonstrated that the reanalysis of large cohorts of women diagnosed with endometriosis from the general population provided peculiar information which is not traditionally associated with the disease and is not informative by standard statistical tests. The illuminating features of the model may improve clinical utility for endometriosis diagnosis and will help to decrease the latency in endometriosis diagnosis.
This article was recently published in the Journal of Personalized Medicine.
Research Source: https://pubmed.ncbi.nlm.nih.gov/35887611/
machine learning AI pelvic pain nutrition genetics dyspareunia diagnosis late engineering endometriosis.