Machine Learning Classifiers for Endometriosis
Nov 5, 2019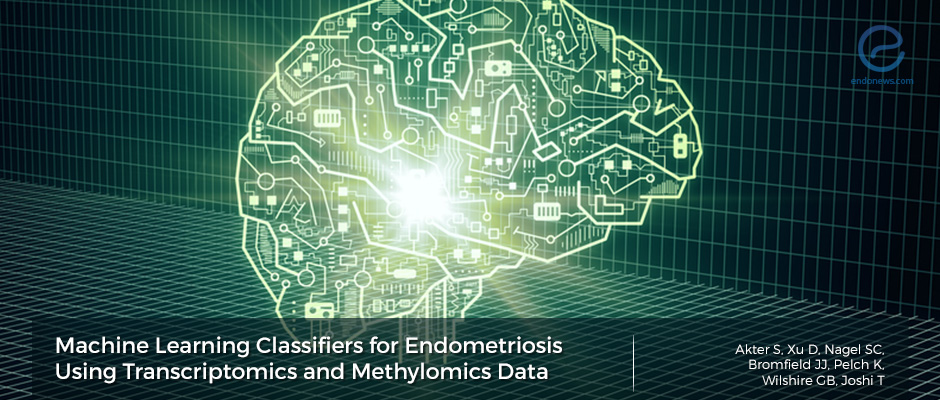
A supervised machine learning method can be a reliable approach for classifying endometriosis
Key Points
Highlight:
- Dr. Joshi group assessed how well the supervised machine learning classifiers perform in classifying endometriosis from the control sample using transcriptomics and methylomics data.
Background:
- Endometriosis is a complicated gynecological disorder that affects about 176 million women worldwide.
- Early intervention is crucial for reducing suffering and expenses related to the disease.
- As endometriosis patients have altered methylome (DNA methylation) and transcriptome (RNA-seq) signatures, identifying these differences could be one way to diagnose endometriosis early.
- Fortunately, the findings of relevant biological signatures from microarray expression or next-generation sequencing (NGS) data have been advanced over the last decades by applying various machine learning tools.
Key points:
- In total, 33 samples for RNA-seq and 77 samples for the methylomics dataset were processed.
- Each dataset has a better normalization method.
- TMM (trimmed mean of M values) normalization performed the best for the transcriptomics dataset.
- Both qNorm (quantile normalization) and "voom normalization" performed the best for the methylomics dataset.
- GLM (generalized linear model) was useful for improving the performance of decision tree models.
- Important endometriosis candidate biomarker genes (eg. NOTCH3) were extracted from these machine learning models.
Conclusions:
- Dr. Joshi group suggests that machine learning classifiers can be trained for creating highly accurate models for classifying endometriosis.
- However, further study in multiple diseases using more data on numerous populations is needed to confirm this result.
Lay Summary
Endometriosis is a complicated gynecological disorder that affects about 176 million women worldwide. It significantly impairs mental and physical quality of life in patients but the etiology is poorly understood. Endometriosis also causes a large economic burden due to loss of workdays and the health-care costs due to hospitalization and medication. Unfortunately, a definitive clinical symptom or minimally invasive diagnostic method is not available and this causes on average 4 to 11 years diagnostic latency. Therefore, early intervention is crucial for reducing suffering and expenses related to the disease.
One of the best ways to reduce diagnostic latency is to develop a minimally invasive diagnostic approach, such as endometrial biopsy. As endometriosis patients have altered methylome (DNA methylation) and transcriptome (RNA-seq) signatures, identifying these differences in DNA methylation and gene expression could be one way to diagnose endometriosis early.
Fortunately, the findings of relevant biological signatures from microarray expression or next-generation sequencing (NGS) data have been advanced over the last decades by applying various machine learning tools. Therefore, in this paper, Dr. Joshi group from the University of Missouri performed if machine learning system, trained with transcriptomics and methylomics data, identifies endometriosis from healthy control samples.
RNA-seq was performed in a total of 38 samples. In the methylomics dataset, a total of 77 samples were processed (35 controls and 42 endometriosis). Since the machine learning training process is a data-driven approach, multiple aspects by various experiments were assessed. First, three different normalization techniques were evaluated. A trimmed mean of M values normalization performed the best for the transcriptomics dataset, and both qNorm (quantile normalization) and "voom normalization" performed the best for the methylomics dataset. This group also found that the generalized linear model was useful for improving the performance of decision tree models. Furthermore, several important candidate biomarker genes were extracted from the machine learning models. For example, NOTCH3 has been identified as a candidate biomarker by all of the methods, which is a protein-coding gene and found to be differentially expressed and downregulated in endometriosis. By comparing transcriptomic and methylomics datasets, the results from the transcriptomic dataset showed higher accuracy.
Based on this result, Dr. Joshi group suggests that machine learning classifiers can be trained for creating highly accurate models for classifying endometriosis. However, further study in multiple diseases using more data on numerous populations is needed to confirm this result. The paper is recently published in "Frontiers in Genetics".
Research Source: https://www.ncbi.nlm.nih.gov/pubmed/31552087
Endometriosis Machine learning Classification Methylomics Transcriptomics DNA methylation RNA-seq Translational bioinformatics