Machine learning tools to predict endometriosis
Feb 10, 2022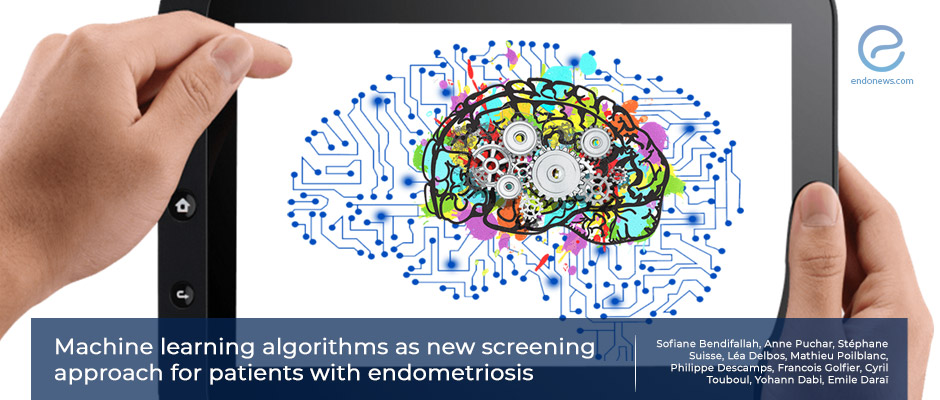
One-click for endometriosis diagnosis
Key Points
Highlights:
- The study gathered endometriosis management and diagnosis methods together, made a machine learning prediction platform, to replace operative diagnosis of endometriosis.
Importance:
- The promising results gave an opportunity to diagnose endometriosis on the first step of the health care system without a delay.
What's done here:
- The data of 8000 patients, suspicious for endometriosis, on a national study platform, have been analyzed.
- After excluding data for missing information, 1126 patient data were used to train the platform; and 608 data have been used as negative control information.
- After the system has been built 100 patients certain for endometriosis diagnosis have been used to validate the program.
- Demographic data, symptoms, and life quality surveys have been taught to the program.
- Appropriate statistical measurements have been used for diagnostic accuracy.
Key Results:
- Machine learning algorithms (MLAs) based on 16 clinical and symptom-based features were found to be appropriate for the prediction of endometriosis.
- The diagnostic efficiency sensitivity was 95% and specificity was 80%.
- The MLA system has been announced as a patient-based diagnostic method as it covers symptoms and life quality questions.
Limitations:
- As the whole setup was done according to the patient statement, there might be some interfering data between endometriosis and control groups.
Lay Summary
The current gold standard diagnostic method for endometriosis is the surgical diagnosis and yet no approaches have been found to replace it.
In the study conducted by Sofiane Bendifallah et al, the authors aimed to use machine learning algorithms to give an opportunity for first step catch of the disease by general practitioners or even patients themselves.
The data of 8000 patients, who are suspicious for endometriosis, on a national study platform have been analyzed. 6266 data have been excluded for missing information and 1126 data have been used to train the platform on endometriosis; 608 data have been used as negative control information. After the system has been built, 100 patients certain for endometriosis diagnosis have been used to validate the program. Demographic data, symptoms, and life quality surveys have been taught to the program. ROC curves, the area under curve calculations, and sensitivity-specificity measurements have been taken under consideration for diagnostic accuracy.
Machine learning algorithms based on 16 clinical and symptom-based features were found to be appropriate for the prediction of endometriosis. The diagnostic efficiency was found as sensitivity and specificity of 95% and 80%, respectively. The Machine learning algorithm system has been announced as a patient-based diagnostic method, as it covers symptoms and life quality questions. Although the setup was laid on patient statements and the validation analyses have been done with a relatively small number of true patients, the study is promising to be used on the first step of the health care to stop the long years of delay on endometriosis diagnosis.
This article was recently published in the journal named "Scientific Reports".
Research Source: https://pubmed.ncbi.nlm.nih.gov/35022502/
machine learning prediction tools Medical research Signs symptoms computing Engineering Biomedical